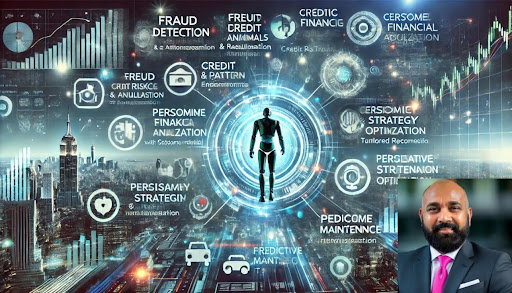
Ardhendu Sekhar Nanda is a leader in the realm of financial technology, renowned for his extensive expertise in Treasury Data Services, Vendor Data Management, and Financial Reporting. With a career spanning over two decades, Ardhendu has consistently demonstrated his ability to drive transformative change within the financial services sector. His work has been instrumental in bridging the gap between technological advancements and business imperatives, earning him recognition as a thought leader and innovator.
Currently, Ardhendu serves as Vice President at a top-tier multinational bank, where he leads Data Transformation in Treasury Operations. His role involves driving strategic initiatives, overseeing data governance, and ensuring the integration of advanced data solutions to enhance operational efficiency and regulatory compliance. His thought leadership is further evidenced by his numerous publications in international peer-reviewed journals where he shares insights on the latest technology trends and developments in Treasury systems and emerging technologies.
Ardhendu has served as a jury member for prestigious awards such as the Globee® Awards and the Stevie® Awards, recognizing excellence in business innovation. His participation in the 2023 Future Business Leaders of America (FBLA) conference and the 2024 Business Professionals of America (BPA) Leadership Conference underscores his commitment to mentoring and empowering future business leaders. Ardhendu's dedication to nurturing the next generation of talent reflects his belief in the transformative power of knowledge and mentorship.
Symbolic machine learning merges advanced AI and machine learning to deliver real-time insights and predictive analytics. This hybrid method, blending symbolic AI's rule-based knowledge with machine learning's pattern recognition, enhances interpretability and transparency. It is especially valuable for financial institutions, improving decision-making, risk management, and customer service while offering benefits like increased predictive accuracy, lower operational costs, and better regulatory compliance. Ardhendu explores its transformative impact on financial operations.
A distinguishing feature of symbolic machine learning is its capacity to elucidate the rationale behind its predictions. Unlike opaque black-box models, symbolic machine learning ensures transparency and accountability, empowering financial institutions to understand and validate AI-driven decisions.
Symbolic Machine Learning in Finance: Applications and Benefits
Symbolic machine learning (SML) combines symbolic AI with machine learning to create interpretable and transparent models. Ardhendu Sekhar Nanda explores its transformative impact on finance, highlighting several key applications:
1. Fraud Detection: SML algorithms detect fraudulent activities by analyzing extensive data in real time. They identify anomalies and potential fraud patterns, helping financial institutions prevent significant losses. Importantly, these algorithms provide audit trails essential for regulatory compliance.
2. Credit Risk Assessment: In banking, SML models assess credit risk by analyzing large datasets to predict default probabilities. This helps banks make informed decisions on credit issuance and interest rates. The transparency of SML models enhances regulatory trust and compliance.
3. Algorithmic Trading: SML enhances algorithmic trading by analyzing market trends and historical data to forecast future movements. These models convert market volatility into actionable trading strategies. Their interpretability allows traders to understand and adapt strategies based on market conditions.
4. Personalized Financial Advice: SML enables personalized financial recommendations by examining customer behavior and financial goals. This tailored advice supports informed decision-making and aligns with individual objectives. The clarity of SML ensures customers understand the basis of the recommendations.
5. Chatbots: SML-powered chatbots improve customer service by handling natural language queries, providing account information, and performing transactions. They offer accurate, explainable responses, enhancing efficiency and customer trust.
6. Market Sentiment Analysis: SML analyzes social media and news data to gauge market sentiment. This helps financial institutions identify trends and adjust strategies. The explainability of sentiment scores improves analysts' confidence in their decisions.
7. Risk Management: SML models are crucial for predicting and mitigating various risks, such as loan defaults and market fluctuations. They analyze multiple risk factors to enable proactive risk management and maintain operational stability.
8. Customer Segmentation: SML algorithms segment customers based on demographics and behavior, allowing for targeted marketing and personalized services. This improves satisfaction and loyalty, with transparent segmentation decisions enhancing credibility.
9. Trading Strategy Optimization: SML models analyze large datasets to refine trading strategies. They identify patterns that inform trading decisions, maximizing returns and minimizing risks. The model's transparency helps users trust and implement strategies effectively.
10. Compliance and Regulatory Reporting: SML automates the analysis of regulatory requirements, ensuring operations adhere to legal standards. The model's explainability facilitates audits and regulatory scrutiny.
11. Predictive Maintenance: In financial institutions, SML predicts IT infrastructure failures by analyzing system logs and performance metrics. This proactive approach reduces downtime and ensures system reliability.
12. Enhanced Customer Service: Beyond chatbots, SML enhances various customer service tools, providing detailed, explainable responses. This improves overall customer satisfaction and loyalty.
In summary, symbolic machine learning revolutionizes finance by integrating symbolic AI with machine learning, creating transparent models that improve fraud detection, credit risk assessment, trading strategies, and customer service. This hybrid approach fosters innovation, enhances risk management, and ensures regulatory compliance, empowering financial institutions with a competitive edge in a dynamic industry.