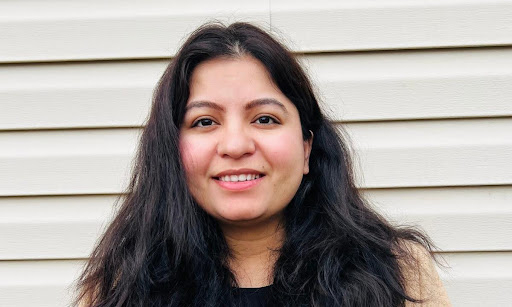
Artificial intelligence is increasingly getting integrated into hiring processes, optimizing tasks such as résumé screening and job matching. However, concerns regarding unintended biases in AI systems have raised critical questions about fairness and equity. Gender bias, in particular, has emerged as a significant issue, with certain algorithms unintentionally reinforcing historical stereotypes by associating specific job roles with particular genders.
Leading platforms such as ZipRecruiter, CareerBuilder, and LinkedIn utilize AI-driven tools to connect job seekers with opportunities. While these tools aim to improve efficiency, they have faced scrutiny for potential biases. In response, LinkedIn introduced additional AI tools to combat bias within its system, demonstrating a proactive approach. Similarly, platforms like Monster and CareerBuilder are exploring strategies to mitigate bias. Despite these efforts, the lack of transparency in AI algorithms still presents challenges in ensuring fair outcomes. A notable example occurred in 2018 when Amazon discontinued its AI-powered recruiting tool after uncovering gender bias within its system.
Research on Mitigating Gender Bias
Amid these challenges, Swati Tyagi, a research scholar at the University of Delaware, in collaboration with Anuj from RingCentral Inc., has introduced innovative approaches to reducing gender bias in AI-driven hiring. Their work, which was recently recognized at the International Conference on Computing, Machine Learning, and Data Science (CMLDS 2024) in Singapore, earned Tyagi the Best Presenter Award from the International Computing and Engineering Association (ICEA).
Tyagi's research, Promoting Gender-Fair Résumé Screening Using Gender-Weighted Sampling, explores methodologies to improve fairness in AI hiring systems. "Our goal was to design a framework that not only identifies bias but actively works to mitigate it," Tyagi explained. The study introduces gender-weighted sampling, a method that adjusts gender representation in training datasets, leading to more balanced and equitable algorithmic outcomes.
Key Findings and Techniques
The research highlights two primary strategies for addressing gender bias in AI hiring systems:
- Gender-Weighted Sampling: This approach adjusts gender representation in datasets to ensure more equitable training of AI models.
- Comprehensive Evaluation: It involves analyzing classifier performance across diverse datasets to identify and correct significant gender imbalances.
These strategies aim to refine hiring processes by promoting fairness, transparency, and improved outcomes.
Broader Implications for Ethical AI
Beyond the CMLDS conference, Tyagi's work was published in the 'International Journal of Information Management Data Insights a leading Q1 Elsevier journal. Her paper, 'Enhancing Gender Equity in Résumé Job Matching via Debiasing-Assisted Deep Generative Model and Gender-Weighted Sampling', introduces a novel debiasing-assisted deep generative model. This approach addresses biases in word embeddings, fostering more equitable vector representations and reducing gender disparities in job classifications.
"AI-driven hiring is not just a technical challenge; it's a societal issue," Tyagi emphasized. Her work underscores the importance of developing responsible AI systems that align with principles of fairness, inclusion, and equality. In an effort to promote further advancements, she has made her research openly accessible and shared the project code on GitHub.
Moving Toward Inclusive Hiring Practices
As AI technologies become increasingly prevalent in hiring, ensuring fairness and transparency is critical. Tyagi's research marks an important step toward mitigating bias and fostering inclusive hiring practices. By prioritizing equity, organizations can leverage AI to create opportunities that reflect the diverse and evolving nature of the global workforce.
In her CMLDS presentation, Tyagi emphasized the societal impact of her research: "AI-driven hiring bias isn't just a technical problem; it's a societal issue. We need transparent, fair AI systems to promote equal opportunities." Her presentation combined technical insight with a clear call to action for the creation of responsible AI systems.
Reflecting her commitment to open research, Tyagi has made her study available as open-access under a Creative Commons license, with the project code hosted on GitHub to support ongoing developments in ethical AI.
As AI technologies continue to evolve across industries, Tyagi's work underscores the critical need for fairness and transparency in hiring systems. By addressing these challenges, her research takes a significant step toward promoting inclusive hiring practices and preventing AI from perpetuating existing social inequities.