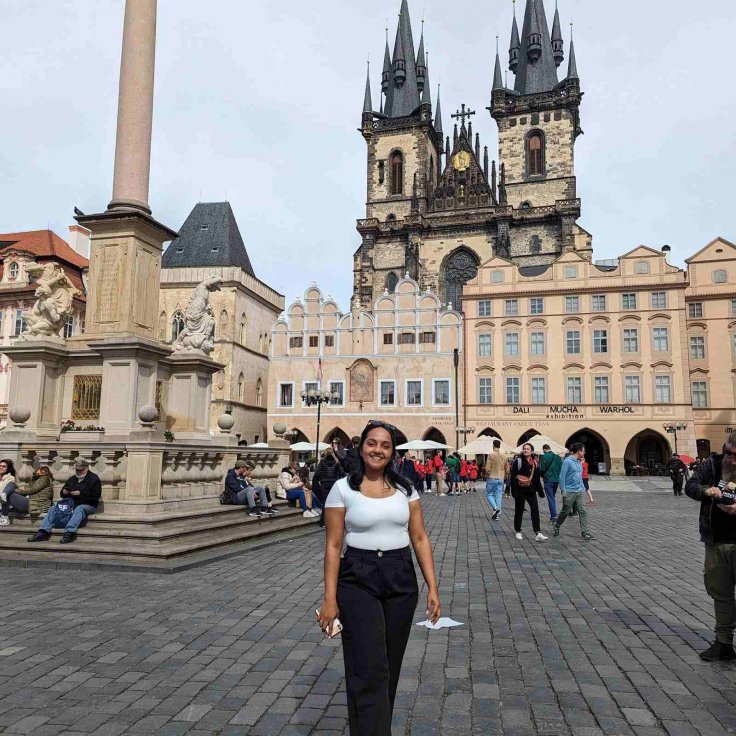
With the development of artificial intelligence, the integration of AI into edge devices is a vital area of concern by semiconductor and technology companies. However, edge AI does not depend on cloud computing but runs AI algorithms in local devices. In this way, efficiency improves while latency decreases and opens doors to increased privacy. Nevertheless, edges AIs are quite difficult to deploy due to power consumption and computational efficiency requirements. Addressing challenges can be solved with ASIC (Application-Specific Integrated Circuit) design with low-power techniques.
A significant advancement in this field has been the creation of innovative low-power design methods for AI accelerators. Professionals like Niranjana Gurushankar have developed techniques that drastically cut power usage without sacrificing functionality. By leveraging architectural optimizations, circuit-level innovations, and software-hardware co-design, the latest AI accelerators have achieved a 25% reduction in power consumption. This reduction is particularly beneficial for battery-powered edge devices, extending their operational life and reducing the overall energy footprint in data centres.
An important factor in optimizing AI for edge applications is ASIC design. Unlike general-purpose processors, ASICs are tailored for specific tasks, allowing for higher efficiency and lower power usage. A critical challenge in AI ASIC development has been ensuring robust verification methodologies, particularly for asynchronous circuits. By developing a novel verification methodology that integrates formal verification techniques with specialized simulation strategies, she ensured the functional and power efficiency of these circuits. This advancement has contributed to the wider adoption of asynchronous circuits in AI hardware, further optimizing power usage and performance.
Beyond power efficiency, AI-driven hardware improvements have also had a significant impact on consumer electronics, particularly in smartphone cameras. She has worked extensively on improving image processing pipelines, identifying and resolving critical bugs in ISP (Image Signal Processor) chips. These efforts have led to a notable reduction in image noise and enhanced colour accuracy, elevating the overall quality of smartphone cameras. The direct impact of these improvements can be seen in higher customer satisfaction, increased sales, and a competitive edge for manufacturers.
A thorough verification library designed for low-power designs is another noteworthy development. By consolidating reusable testbenches, verification IPs, and power analysis tools, engineers have refined the verification process. This initiative has not only reduced verification time by an average of 10% but has also encouraged best practices across teams, ensuring consistent and high-quality low-power designs.
Low latency is another crucial factor in edge AI applications, particularly for real-time services such as video streaming and autonomous systems. Optimization of data flow and processing pipelines has led to a 20% reduction in latency for real-time streaming services, significantly improving user experience. This optimization has not only enhanced service quality but has also contributed to subscriber growth, demonstrating the impact of hardware and software co-design in AI applications.
The growing reliance on AI in hardware design has also emphasized the importance of security verification. With cyber threats on the rise, engineers have led initiatives to develop security verification methodologies tailored for ASICs. By integrating formal verification, fault injection testing, and side-channel analysis, these methodologies have enhanced the security posture of AI-powered edge devices. As AI adoption continues to expand, ensuring robust security verification will remain a critical priority.
The use of AI in hardware verification is becoming more popular, automating tasks like test creation, bug detection, and performance optimization. These new trends are influencing the direction of AI in the future. Additionally, agile and DevOps practices are being increasingly adopted in hardware development, fostering faster iteration cycles and improved collaboration across teams.
Niranjana Gurushankar believes that formal verification, emulation platforms, and investment in automation will improve the efficiency of AI hardware development. Because of the rapid advancements of semiconductor technology, engineers need to be aware of the latest developments by engaging in R&D and knowledge-sharing initiatives.
With AI at the edge once more poised for explosive growth, those investing in power-efficient ASIC design and robust verification techniques will stay ahead in this technology revolution.