When the Coronavirus outbreak transformed into a pandemic, government and civic authorities followed the traditional way of quarantine, test, detect, and treat. In Wuhan, China where the virus originated, authorities followed the pattern and were successful. Singapore too tailor-made a similar process and saw positive results.
All of them managed to be successful while the rest of the world plunged into chaos. To keep people away, isolation, robot dogs, drones, and all other means have been used but with little success. But despite that, all three models, which were initially successful, witnessed a spike in cases. And while the success was correctly attributed to those named, superspreading hotspots quickly transmitted hundreds of cases in a day.
In Singapore, migrant workers' dormitories became clusters while in Beijing, China, a central food market became a hotspot. Similar cases emerged in the U.S., Australia, South Korea, and other countries around the world.
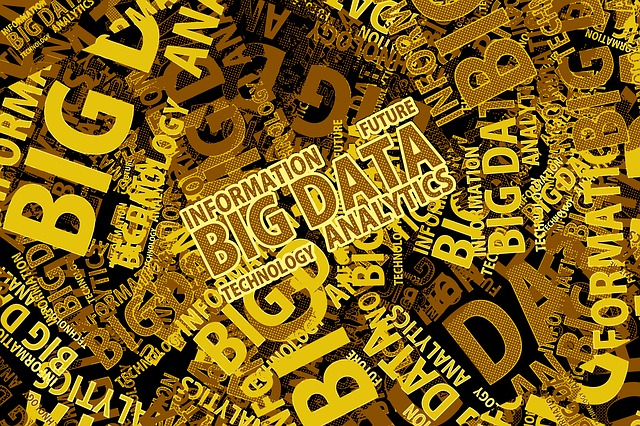
Increasing Number of Hotspots
Already over six months have passed in dealing with the virus and the global infection has crossed a 13-million mark but governments are still in dilemma over whether to impose lockdown and curb the spread or open up businesses to save the economy. In such a case, a prediction model can be used to curb the spread while keeping businesses open.
Singapore University of Technology and Design (SUTD) professors — Roland Bouffanais and Sun Sun Lim — have suggested that governments need tools to assess and predict riskiest places in cities. They believe big data analytics can be a tool governments can harness.
"Big-data studies of human mobility need to be combined with epidemiological models. And the demographic profiles of people coming into contact at any particular location need to be included," they said in a Nature commentary, published on July 10.
Hotspots or Superspreaders?
The hotspots are generally crowded places where human interaction is almost impossible to avoid. Such places like markets, shopping districts, restaurants, and hospitals. In those places, factors like duration of contact, physical proximity, and environmental conditions greatly attribute to the increased risk of spread. Then, there are people who don't comply with safety measures.
Barring those, there are also factories, warehouses, and religious gatherings that have emerged as superspreaders. For example, meat processing units in the U.S. Canada, Germany, Spain, Australia, and in many other countries, have witnessed outbreaks while in Delhi, India a religious congregation — Tablighi Jamaat — reported over 4,000 confirmed cases.
Similarly, cruise ships too have been such hotspots. In Singapore, about 95 percent of the cases have been reported from poorly ventilated and overcrowded workers' dormitories. Hong Kong also saw a similar outbreak in apartment buildings in 2004 for Severe Acute Respiratory Syndrome (SARS).
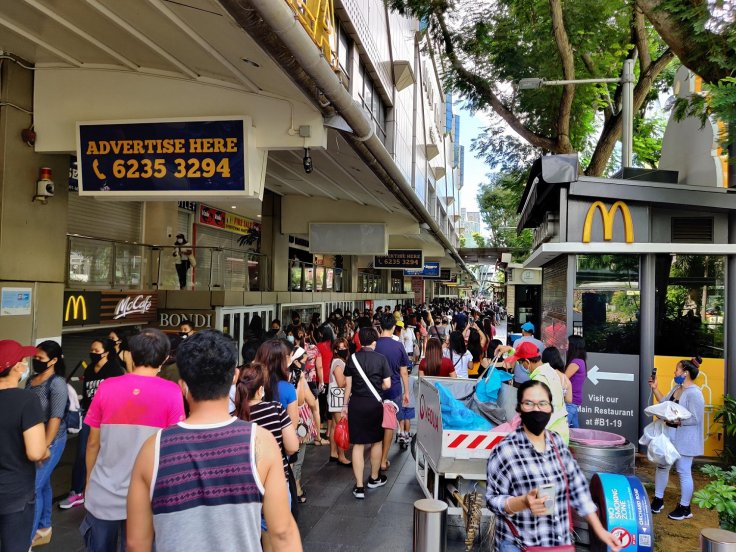
How to Stop Such Locations from Becoming Hotspots?
Contain, detect, and treat model may have worked in some cases but in today's time, city authorities cannot stop the flow of people. As the dilemma continues over stopping the spread with lockdown measures or opening up economy, the authorities need a prediction model that will be helpful, pointed out Bouffanais and Lim.
While authorities are already using contact tracing apps and wearable devices to efficiently detect the spread, Bouffanais and Lim believe a base map is required to "represent the main flows of people throughout a city". That will be able to help in determining key places where people travel like schools, bus stations, and shopping centers and their timings.
According to them, in smart cities like Singapore, a network of CCTVs and cameras can be reconfigured to track the density and mixing of people while built-in barometers in smartphones can follow vertical movement in office buildings. Harnessing the power of social media will also help in creating a predictive model as users post almost everything on their timelines.
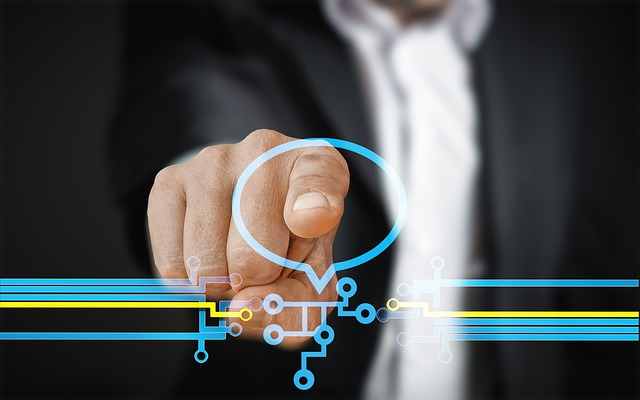
"We'll need to deploy data analytics tools in schools, hospitals, and workplaces to make sure that we are screening people appropriately as they come in the door of those settings," Dr Julie Swann, professor and department head of the Fitts Department of Industrial and Systems Engineering at North Carolina State University, told HealthITAnalytics.
She added that surveillance needs to be nearer to real-time and at a local level. "If you look at the US's influenza-like illness surveillance, it's great at the national level, and it's pretty good at the state level. But it's not very good at community levels," she said
Privacy Concerns
However, with such mass tracking to create a predictive model of virus spread, data privacy concerns are real and such instances have already been seen in France, Italy, India, and in other countries around the world.
Dr Swann said that data privacy must be made top-priority while creating such models of mass surveillance. She added that strategies must be centered around proactive data tracing at the base level.
"With these data and technology changes, we'll need to deal with potential privacy concerns – whether those concerns are specific to healthcare information or more general apprehensions," she noted.