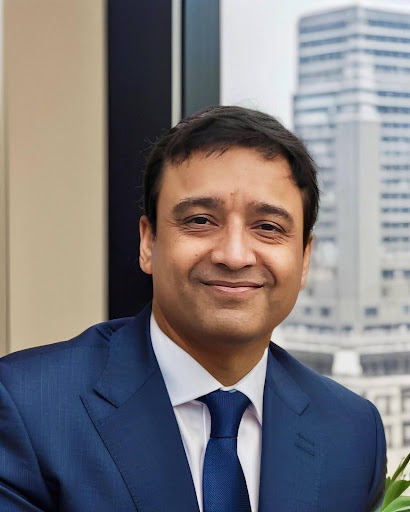
As the financial world becomes more complex, the effectiveness of traditional credit risk models is increasingly challenged. Over time, banks have adopted Artificial Intelligence (AI) and Machine Learning (ML) algorithms to enhance their credit risk management processes.
Sanjay Moolchandani, a distinguished Risk Technology Expert in Banking with close to two decades of rich experience, examines the adoption of AI in banking risk management, giving banks and financial institutions a competitive edge. Sanjay has pioneered and guided technology strategies in banking and is optimistic about the future of AI in banking risk management. His extensive experience and numerous accolades, including a 2024 Global Recognition Award for his contributions to the banking industry and the prestigious Global Leader in Banking & Risk Technology Excellence Award, underscore his authority and insight in this field. Additionally, Sanjay is a Senior Member of IEEE, a distinction held by only ten percent of its members, highlighting his commitment to technological advancement.
The Power of AI and ML in Credit Risk Management
AI & ML models can analyze vast volumes of data with unparalleled speed and accuracy. These advanced models can uncover complex relationships and interactions between variables, leading to more accurate risk assessments and predictive insights. Additionally, these models enable continuous learning and adaptation to changing market conditions, allowing for real-time risk monitoring and proactive risk mitigation strategies.
"AI and ML models are game-changers in how we approach credit risk, providing insights and efficiencies previously unimaginable," says Moolchandani, drawing from his extensive experience. His contributions have transformed technology insights for banking and risk management.
Over the years, banks have adopted several models for credit risk management. Logistic Regression is used for binary classification, predicting credit default. Decision Trees split data into subsets to identify key risk factors. Random Forests combine multiple decision trees to enhance accuracy and handle large datasets. Gradient Boosting Machines correct errors from previous trees, capturing complex patterns with high accuracy. Neural Networks learn complex patterns, suitable for managing large datasets and non-linear relationships in credit risk tasks.
"Each of these models brings unique strengths to the table, allowing us to tailor our approach to the specific needs of our credit risk assessments," Sanjay Moolchandani explains. Sanjay has championed the use of AI and ML in banking risk management.
The Need for Explainable AI
Despite the robustness and accuracy of AI and ML models, they often operate as "black boxes," making it difficult to understand how they arrive at their decisions. In credit risk management, this can lead to concerns about fairness, bias, and ethical implications. Therefore, there is a growing need to ensure transparency, trust, and accountability in decision-making processes.
Explainable AI (XAI) addresses this need by demystifying AI models, enabling banks to adhere to regulations more effectively and build trust with stakeholders. XAI helps identify and mitigate potential biases, ensuring fair treatment for all borrowers and ethical lending practices. It facilitates the identification of errors and refinement of AI models, empowering users and decision-makers.
"Explainable AI allows us to not only understand and trust AI decisions but also to refine and improve these models continually," Moolchandani adds.
Key Features and Techniques in Explainable AI
Several features and techniques enhance the transparency and accountability of AI systems. Local Interpretable Model-agnostic Explanations (LIME) approximate black box models with interpretable ones to explain predictions. SHapley Additive exPlanations (SHAP) use Shapley values from game theory for local explanations. Feature Importance ranks input features by their impact on predictions. Decision Trees provide clear decision paths, making them inherently explainable. Counterfactual Explanations suggest changes in features to achieve desired outcomes. Partial Dependence Plots (PDP) show the marginal effect of features on outcomes. Global Surrogate Models are interpretable models trained to mimic black box predictions.
"These techniques make AI models more transparent and actionable, enabling us to communicate the reasoning behind decisions effectively," notes Moolchandani.
Challenges in Implementing Explainable AI
Integrating Explainable AI (XAI) into credit risk management faces several challenges. Balancing the accuracy of complex AI models with their explainability is difficult. Ensuring data privacy and security compliance, such as with GDPR, is essential when handling sensitive data. Incorporating XAI into legacy systems without disrupting operations poses another hurdle. There is also a skill gap, with a shortage of experts skilled in both AI and explainability methods. Lastly, ensuring that XAI models provide unbiased and fair explanations is critical for maintaining trust and fairness.
"Balancing the complexity of AI models with the need for transparency and explainability is crucial. Additionally, ensuring compliance with data privacy regulations and integrating XAI into existing systems without disruption are significant hurdles," says Moolchandani.
Future Trends in Explainable AI for Credit Risk Management
Future XAI trends include enhancements in AI understandability along with increasing regulations and data privacy. Hybrid methods combining machine learning with traditional statistical approaches will balance accuracy and explainability. Open-source platforms will democratize AI tools, empowering smaller financial firms. Additionally, a growing focus on ethical AI will underscore the importance of XAI in identifying and mitigating unfair automated decisions.
"We will see increasing regulatory restrictions around AI and data privacy, which will drive the adoption of Explainable AI in financial institutions," predicts Moolchandani. Sanjay Moolchandani's work in this area has been exemplary. He has developed innovative solutions using AI & ML technology and has pioneered the adoption of XAI.
Final Thoughts by Sanjay Moolchandani
"Explainable AI is essential for ensuring that AI models in credit risk management are transparent, fair, and accountable. Financial institutions can make informed decisions by understanding AI models through XAI while adhering to ethical and regulatory standards. As we continue to innovate and integrate these technologies, the focus must remain on building systems that are not only powerful but also trustworthy and fair," concludes Sanjay Moolchandani.