Despite extraordinary efforts from many of the leading names in the tech and automotive industry, we haven't seen a fully autonomous car. You can buy a car which can automatically brake for you when it anticipates a collision or one that helps you keep in one lane and even Tesla Model S, which has the autopilot setting that mostly handles highway driving. But almost every one of these carmakers struggles to make self-driving cars work properly.
But it was claimed recently that a well-known self-driving car dataset for training machine-learning systems, used by thousands of students to build an open-source self-driving car which includes critical errors and omissions, as well as missing labels for hundreds of images of bicyclists and pedestrians.
The machine learning models are equally good as the data on which they are trained, when researchers at Roboflow, which writes boilerplate computer vision code, checked the 15,000 images in Udacity Dataset 2, they found issues with 4,986 images.
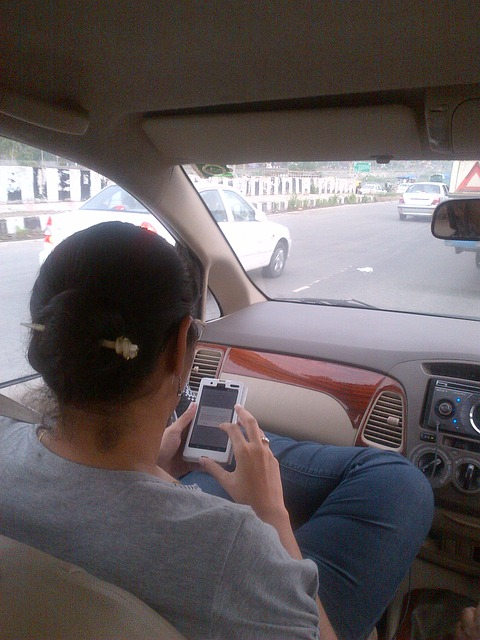
Self-car driving car issues
The finding, which was published by Roboflow founder Brad Dwyer on Tuesday, February 11 showed that there were thousands of unlabeled vehicles, hundreds of unlabeled pedestrians and dozens of unlabeled cyclists. In the blog post, Dwyer mentioned that "We also found many instances of phantom annotations, duplicated bounding boxes, and drastically oversized bounding boxes. Perhaps most egregiously, 217 (1.4 percent) of the images were completely unlabeled but actually contained cars, trucks, street lights, and/or pedestrians."
In terms of artificial intelligence behind self-driving cars, junk data could literally lead to deaths. While describing how unreliable data propagates through a machine learning system, Dwyer stated that even though it is believed that machine learning can bring changes in self-driving cars, it should be noted that "With great power comes great responsibility; a poorly trained self-driving car can, quite literally, lead to human fatalities."
As reported by Sophos, Dwyer explained that "You give it a photo, it makes a prediction, and then you nudge it a little bit in the direction that would have made its prediction more 'right'. Where 'right' is defined as the 'ground truth', which is what your training data is." In addition, he also mentioned that if the training data's ground truth is wrong, then the model will learn the wrong thing.
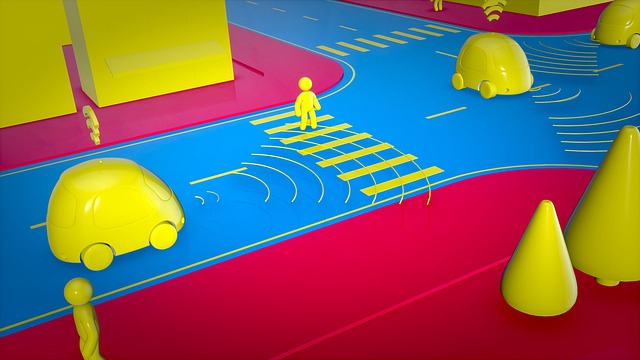
What could get wrong with self-driving cars?
It should be noted that Roboflow has fixed and re-released the Udacity self-driving car dataset in a number of formats, while Dwyer urged to consider switching to the updated dataset to those who were training a model on the original dataset. Since Dwyer hasn't looked into any other self-driving car datasets, it is hard to describe how much bad data is sitting at the base of AI training.
In 2018, 49-year-old Elaine Herzberg was killed by a self-driving car as she walked her bicycle across a street in Tempe, Arizona. After that incident, the responsible company, Uber said that her death was likely caused by a software bug in its self-driving car technology.
But as per Dwyer, bad data quality had nothing anything to do with the tragic crash. As per a federal report released in November 2019, the self-driving Uber SUV involved in the crash couldn't figure out if the woman was a jaywalking pedestrian, another vehicle, or a bicycle, and it failed to predict her path's trajectory, while its braking system wasn't designed to avoid an imminent collision.
Udacity response
Udacity created this dataset years ago as a tool purely for educational purposes, back when self-driving car datasets were very hard to come by, and those learning the skills needed to develop a career in this field lacked adequate training resources. At the time it was helpful to the researchers and engineers who were transitioning into the autonomous vehicle community. In the intervening years, companies like Waymo, nuTonomy, and Voyage have published newer, better datasets intended for real-world scenarios. As a result, our project hasn't been active for three years.
We make no representations that the dataset is fully labeled or complete. Any attempts to show this educational data set as an actual dataset are both misleading and unhelpful. Udacity's self-driving car currently operates for educational purposes only on a closed test track. Our car has not operated on public streets for several years, so our car poses no risk to the public.