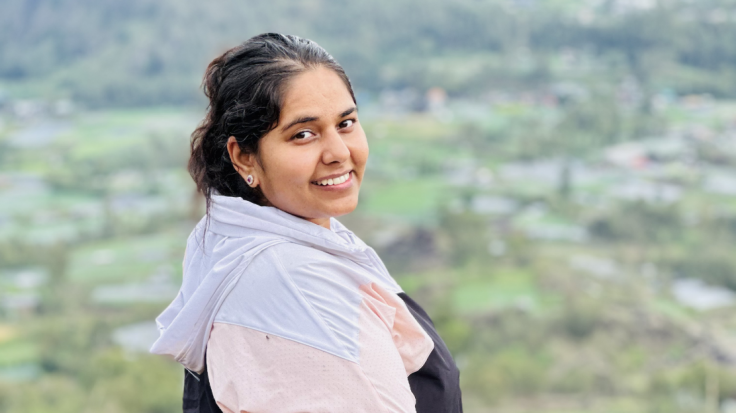
The reliance of financial institutions on business intelligence (BI) is seeing a rise. It is done for comprehending complex markets and making informed decisions. In the current timeline, the integration of real-time analytics into financial strategies is not a luxury anymore. This shift is being led by professionals like Simran Sethi, whose expertise in BI and data science is leaving imprints in the financial sector.
The financial services industry has long relied on data to drive strategy, but the speed at which decisions must be made has increased dramatically. This is where BI and real-time analytics come into play. By providing up-to-the-minute data insights, financial firms can respond more quickly to market shifts, improve risk management, and enhance overall decision-making.
For Sethi, an accomplished Data Scientist and Financial Technology Leader, this transformation is both a career achievement and a passion. Her journey in real-time analytics began with a notable achievement at the McKinsey Analytics Hackathon, where her team developed an end-to-end real-time analytics prototype tailored for the financial services sector. The project integrated big-data pipelines and predictive modeling to enable dynamic financial decision-making, and its success highlighted the power of real-time insights in reshaping traditional financial operations.
Over the years, the professional has continued to build on this foundation. She has researched on financial data lakes, which addresses challenges around data ingestion, governance, and analytics in large-scale financial institutions. Her research provides a comprehensive framework for building and governing data lakes, with a focus on enabling near real-time analytics.
At her current workplace, she has been instrumental in driving key results. She stated, "I introduced real-time dashboards and BI reporting that reduced manual data consolidation efforts by 50–60%." Moreover, by implementing near real-time monitoring for sales pipelines and investment portfolios, her firm has seen empowered decision making from proactive, data-driven decisions.
Sethi has also led several major projects, including the development of an ensemble-based model for fraud detection at the McKinsey Analytics Hackathon. "Although a prototype, the solution demonstrated high accuracy and offered an actionable framework for financial institutions looking to spot anomalies earlier than traditional systems," she added. Her work on a financial data lake consolidates data from multiple systems into one platform, improving reporting and forecasting.
The fraud detection model has improved detection rates by 15-20%. In addition, the increased accessibility of BI tools has led to greater adoption across non-technical staff, boosting the overall effectiveness of data-driven decision-making within the company.
However, there were some challenges like fragmented financial data silos. Many teams at her previous workplace in e-commerce used incompatible systems, making it difficult to obtain a unified view of the organization's financial health. To address this, an enterprise data lake approach was implemented that consolidated the siloed data under a single governance layer, ensuring consistency and data accuracy across the organization. Ensuring compliance with financial regulations was another challenge. This was addressed by using role-based access controls and data masking to protect sensitive information.
By looking at the journey of this professional in the field of finance, certain things are clear as per the future is concerned. For instance, financial business intelligence will increasingly rely on self-service analytics, enabling non-technical professionals to create and interpret dashboards. The combination of AI and BI will automate tasks like data cleaning and anomaly detection, freeing analysts to focus on strategy.
As real-time data becomes more common, "lakehouse" architectures will merge the flexibility of data lakes with the reliability of data warehouses, speeding up real-time analytics and compliance. Finally, ethical standards in data use will be essential to maintain trust with regulators, investors, and customers.